Lifelong Learning
Tags | AdvancedCS 330 |
---|
Review of methods
multi-task learning
is when you learn to solve a bunch of tasks
meta-learning
is when you use examples of tasks and learn a way to solve a new task quickly.
In the real world, however, things are more sequential. Given a set of tasks fed to us, can we get better?
The different setups
Unfortunately, lifelong learning is a very active field and the definitions haven’t solidified.
- Discrete vs continuous: do you get different, labeled tasks, or do the tasks slowly change?
- Level of data storage: do we get access to all the prior data? Or is this not possible/not allowed? On one end of the sepctrum, you have a large replay buffer. On the other end, you have a singular point drawn from a mysterious black box and it disappears after training.
- Desired performance: do we only want best performance on the current task, or do we want good performance on all prior tasks?
- Task order: is it predictable? Random? Is it a curriculum? Is it adversarial (like email spam filtering).
- an
iid
setting is where you draw shared distribution. For curriculums and adversarial, however, this may not be the case.
- an
- Data exposure: do we expect the model to generalize zero-shot to the new data, or do we provide some support data?
Other terms for lifelong learning include online learning, lifelong learning, continual learning, incremental learning, and streaming learning.
What do you want?
We want to minimize regret
, which is the cumulative cost of the learner as it goes through the data, verses a learner that takes all the data and fits things all at once.
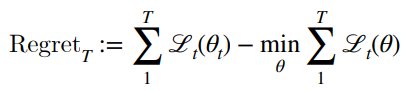
Now, it may well be possible that the regret is negative; if you have highly temporally correlated tasks, a near-sighted model may do better on each individual task as compared to the whole data.
What regret do we want?
Linear regret is trivial. If the regret grows linearly, it means that we aren’t improving at all; we keep on messing up the same degree every time. This can be as stupid as outputting a constant prediction, but it could also mean that the model is learning from scratch for every task somehow.
Therefore, we want sublinear
regret.
Positive and negative transfer
forward transfer
is having previous tasks affect your future tasks. Positive forward transfer means that past tasks allow future tasks to perform better than learning-from-scratch. Negative forward transfer means that opposite.
backward transfer
is having current tasks affect your previous tasks. Positive backward transfer means that past tasks perform better after seeing more data. Vice versa for negative.
Ideally, you want both positive forward and backward transfer.
Basic approach
Follow the leader
This is very easy. Just store all the data, and train on it.
- very strong performance (as long as it isn’t adversarial)
- computationally intensive and memory intensive
SGD
For every data point you observe, take a gradient step in this direction (or a few)
- very computationally cheap and requires very little memory
- subject to negative backward transfer (catastrophic forgetting)
- learning is slow because of SGD being stochastic
Variants
You might only use the current task data but continue to use some sort of model from the past, like a Q function
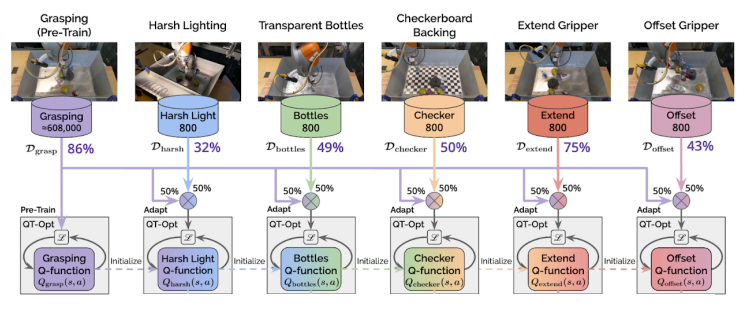
Improving from the basics
Improving SGD
Can we improve SGD to avoid negative backward transfer? Here’s one idea
- store small amounts of data per task in memory
- For the current optimization, compute gradients for each of these tasks. Then, generate a gradient for the current task such that the current gradient has a non-negative inner product with all other task gradients.
We can solve for this constraint using quadratic programming.
Meta-learning perspective
If we assume that we get a little bit of information about the task beforehand, then we can just reframe it as an online meta-learning task. Each task is a meta-task, and the small amount of data is the support example.
You can think of this as follow the meta-leader
, where you meta-train on the existing data and run update procedure. This removes the assumption that and all are the same. However, this can still suffer from OOD tasks. For better performance, optimization based approaches can be helpful.