Gaussian Channel
Tags | ContinuousEE 276 |
---|
Gaussian Channels
The gaussian channel is just a continuous RV with an added noise vector
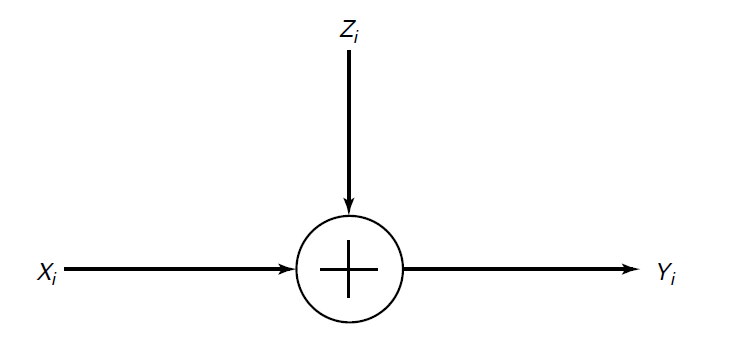
Of course, if weren’t constrained, the capacity is arbitrarily large because you can essentially make as large as possible to drown the noise out. So, we are interested in situations where the has a certain limit:
where is the power limit.
The channel
We make a power-constrained channel as follows
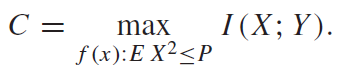
And we can use the chain rule of MI and the knowledge that to yield the following derivation
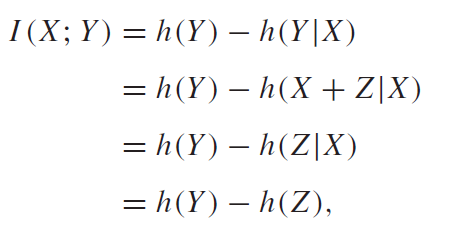
We know the closed form expression for .
Now, we continue by realizing that . We know that because they are independent and . Now, this is actually really nice. We know that a gaussian maximizes this second moment, so . But is this gaussian achievable?
As it turns out, yes! The sum of two independent gaussians is gaussian, so we can just use the maximizing distribution for , which is the gaussian with variance . If we add this to , we get the maximizing distribution for . In this case, we have
And this is the capacity of a gaussian channel! We call the the signal to noise ratio
, and we see how the capacity depends on it.
Quantized Gaussian
You might let be a binary signal. This gets you a quantized channel with the being a bimodal gaussian. This reduces the capacity (still by symmetry, the should be uniform).
You might further choose to quanitize the output . At this point, you have created a BSC with crossover probability , which is a function of the power limit and the noise of . You calculate this by taking the CDF.